How is AI used in Aquaculture?
Aquaculture is the breeding and harvesting of aquatic creatures. We’ll talk about companies that focus on fishes. The usage of AI in aquaculture has streamlined and expedited the daily tasks of fish farmers.
In the past, fish farmers had a challenge of estimating fish population and harvests. They relied on laborious and prone to error traditional approaches to tackle it. But, with the growth of the aquaculture industry, there is a higher need for precision. Luckily, Artificial Intelligence (AI) has emerged. AI is a game-changer for fish farmers in Singapore.
Usage of AI in Farms:
-
Estimate fish population:
Farmers will record videos of fish swimming across a net, with a white canvas underneath. The videos will then be ran on a software for the AI to detect and estimate the number of fishes for the farmers.
-
Estimate the number of harvested fishes:
Farmers will first need to lay out the harvests. Then, they have to open the fish detection app, take a photo/video or select one from their gallery, and let the app run. The AI algorithm will count the fishes for them.
With AI, farmers now have a modern and innovative tool that helps with their farm operations. This technology enhances accuracy, efficiency and promotes sustainability & responsible fish farming practices.
Why is AI used in Aquaculture?
Cost Savings
The use of AI to count fish reduces farmer’s reliance of manual labour for fish counting tasks. It also saves their valuable time and effort. In turn, farmers can redirect their attention towards other essential farm activities. This reduces labour costs and enhances efficiency and productivity in farm operations.
In the long run, the benefits that farmers get far outweigh the cost of purchasing a fish counting AI system.
AI Automation of the Fish Counting Process
Farmers used to do manual fish counting. It was time-consuming, labour intensive and prone to errors. This led to inaccurate fish population or harvest estimates.
AI makes the fish counting process completely automated. AI will count the number of fish. As a fish farmer, you won’t have to do this manual process. You will get more reliable results compared to the traditional manual processes.
Contactless & Quicker Process
Implementing AI for fish counting has increased the speed of obtaining results. You don’t need physical contact. There’s no longer a need to manually track and identify each fish. Without the need of a fish farmer to physically be there. It reduces stress & the risk of harm to the fish, leading to a quick and less disruptive fish counting process.
Larger Customer Base & Positive Reputation
By using AI, farmers can showcase their commitment to sustainable and high-quality practices. It also reflects a proactive approach towards minimising environmental impact and resource wastage. This helps to attract a larger customer base. You can foster a positive reputation in the market.
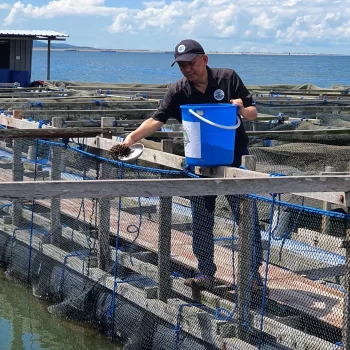
Sustainable Development
Accurate Stock Management
AI fish counting allows farmers to improve fish stock management accuracy. Farmers can account for all fish populations within their farms. This helps in making informed decisions on their stocks and harvests. It also improves farm productivity and profitability.
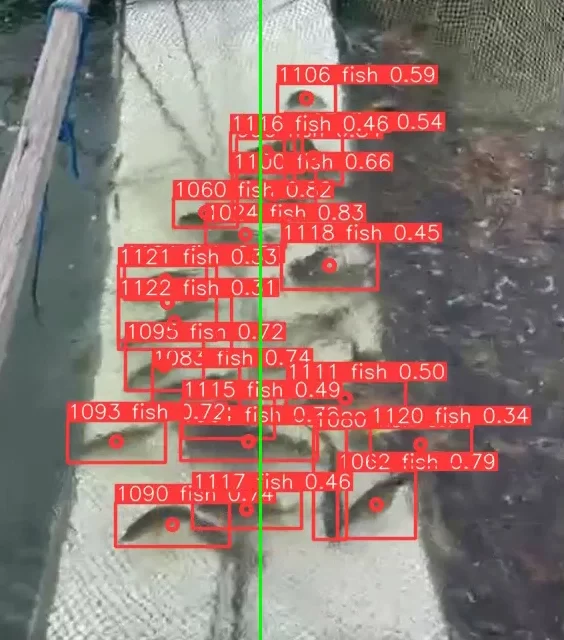
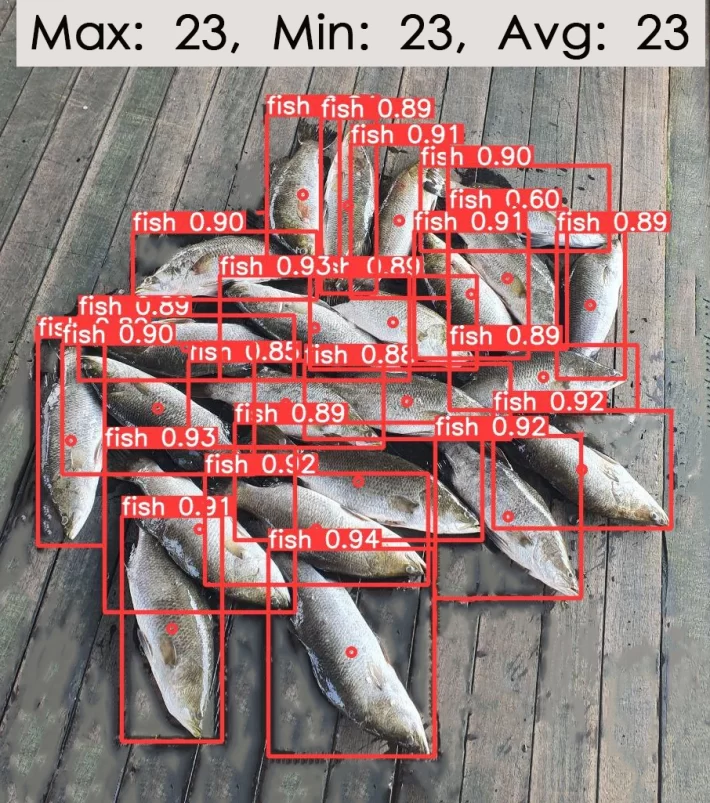
What has MWI done to help?
MWI has a lot of expertise in the fish farming and AI software industry. We understand the important of AI in the aquaculture industry in Singapore. We also acknowledge the challenges and limitations that farmers face. To address them, we have developed an AI fish counting model from scratch. For fish farm businesses to use.
Even MWI’s sister company, The Fish Farmer, uses our AI model to assess its fish population and harvest.
FAQs
There are a couple of methods but we are only going to name and discuss a few here.
Hand/Manual Counting of Harvests
Fish farmers would count the fish one by one. This method needed a lot of time and effort, especially in large farms with high number of fish harvests. It is also more prone to human errors.
This method has inaccurate estimated. This inaccuracy impacts resource decision making in fish farms. This in turn affected the productivity on the farm.
Visual Observation of Live Fishes
Farmers will stand by the pond and observe the fishes, to gauge the number of fish present. They draw conclusions based on their judgement and expertise. This method is simple, inexpensive and based on farmer observation skills and attentiveness. But, challenges arise when fish swim in schools or overlap one another. It makes it challenging to count each individual fish.
Mark-Recapture of Live Fishes
Farmers fish for a certain number of fishes and mark them before releasing them back into the pond. After a period of time, farmers will fish again. They will then examine them for the presence of markings. Farmers compare the proportion of marked fish in the second sample to the first sample. This allows them to estimate the total population size of their fish farm.
Farmers use tags or dye to keep the fish marks intact when they recapture them. This method is pretty accurate for estimating fish population size. But, there are also limitations to this. There is a possibility that the fish may lose their tags/dye marks when recaptured. It would make it difficult to identify the marked fishes during the second stage.
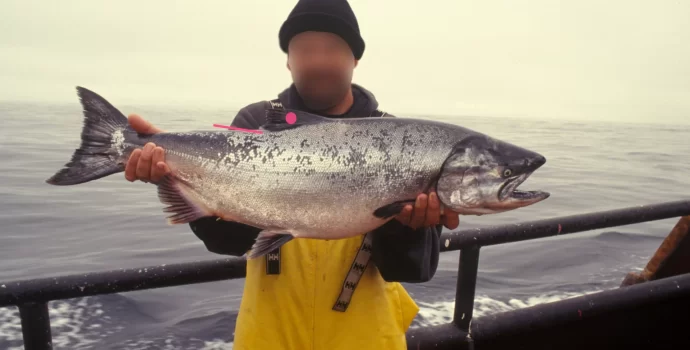
Conclusion
Working with The Fish Farmer, MWI employs vision AI in aquaculture in Singapore to count fish population with Object Detection and Tracking Algorithm.
Object Detection is the process of identifying and localizing objects of interest in an image or video frame.
It has two primary tasks:
(a) object localization, which determines the precise location of an object within an image, typically through bounding boxes, and;
(b) object classification, which assigns a label or category to the detected object.
Object Tracking is the process of locating and tracking objects in a video or image sequence. It is the detection of the object in each frame of the video and determining its position, size, and motion. There are several popular algorithms available. For this exercise, we use State-of-the-Art (SOTA) YOLOv5, YOLOv8, YOLO-NAS technologies.
Custom AI Model
This part of the blog gives an overview of how the fish counting vision AI model works from a layman’s perspective. Let’s begin.
At a high level, there are 3 steps in creating a custom AI model to do fish counting. We call these steps; training, validation and testing. This process is iterative in nature. More training, validation, and testing lead to a more accurate model.
1. Training Of Computer Vision AI Model
Imagine you are teaching a child to know the alphabets using flashcards. You would show him many variations of each alphabet. There will be variations of colour, size, shape, orientation and more. Showing many different versions of letters helps the child learn what each letter is made of. This is AI at its very core.
In this example, the “flashcards” or dataset will be the fishes in the local fish farm. Fish comes in varying sizes, colours, shapes, and have unique behaviours in different lighting and water conditions. More images of these fish in various states improves the model’s fish prediction accuracy. Training is the term used to describe the process of teaching the AI algorithm (the ‘brain’) to identify a fish.
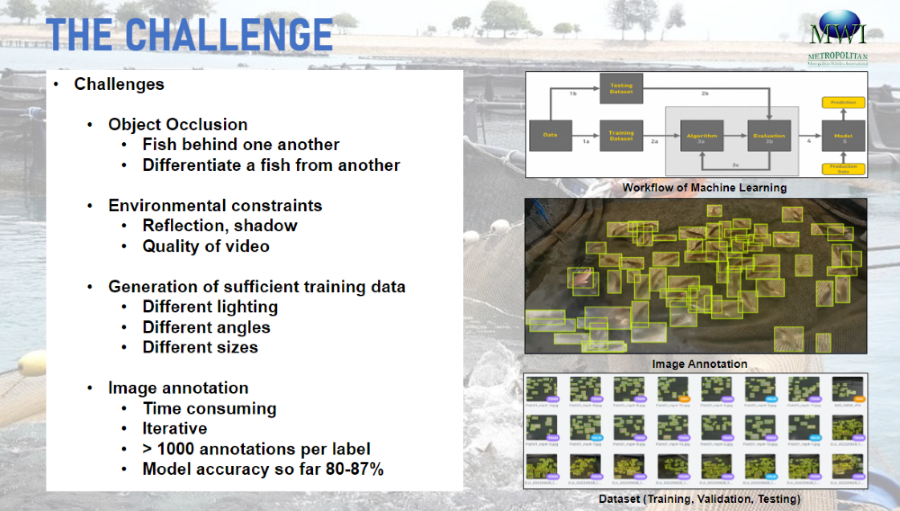
2. Validation of Computer Vision AI model
Training alone is not enough. A child may have a superb memory and know each flashcard by heart without knowing the subject matter. The next step is validation. What a past year exam paper reveals about a student’s ability is what validation does to an AI model.
During validation, we give the model unseen data. For example, “past years’ exam papers”. We then compile the results. How well the child scores will give us a sign of how much he knows his subject beyond mere memory. Say that the model has high mAP (‘score’) for training but low mAP for validation. This is a sign that the model has not learnt and is memorising the ‘flashcard’. In AI lingo, the model is not a generalised model. This meant that there is a need to have more training with different variations. Now, the entire cycle repeats itself.
3. Testing of Computer Vision AI model
The real test of a child’s ability is when he finally sits for his examination in school. The test papers are completely unknown to him beforehand. During this time, the real test of his knowledge (or lack of) reveals itself. This process is called testing of the AI model. In general, a high mAP for validation will show a high mAP for testing.
These three steps outline the creation process of the AI in aquaculture.
Here's a video of how AI is used in a local fish farm
The Singapore Aquaculture Plan is a strategy to grow the local fish farming industry. It aims to use technology for better and more sustainable farming. The plan wants to make Singapore less dependent on imported fish. It supports farmers with funds, research, and training. This helps to increase local fish production and ensure a stable food supply.((“The Singapore Aquaculture Plan.” Our Food Future. Accessed October 9, 2023. https://www.ourfoodfuture.gov.sg/uplifting-aquaculture-industry/sg-aquaculture-plan/.))
In Singapore, AI helps in fish farming. It tracks fish health and water quality. Cameras and sensors collect data. AI analyses this data. It predicts diseases and helps in feeding. This boosts yield and lowers costs. AI also plays a big part in helping achieve the Singapore Aquaculture Plan!((Cue. “Offshore Fish Farms May Need to Step up Sustainable Practices to Protect Marine Life, Water Quality.” The Straits Times, July 15, 2023. https://www.straitstimes.com/singapore/offshore-fish-farms-may-need-to-step-up-sustainable-practices-to-protect-marine-life-water-quality.))